Noise
Noise in machine learning refers to irrelevant or meaningless data or distortions in a dataset. Noise can come from errors in data collection, transmission, or processing and can adversely affect the performance of a machine learning model.
Dealing with Noise in Machine Learning
Effective handling of noise is crucial for building robust machine learning models. Techniques to reduce noise include data cleaning, outlier detection, and the use of noise-robust algorithms. Recognizing and mitigating noise can lead to more accurate models and better generalization to new data.
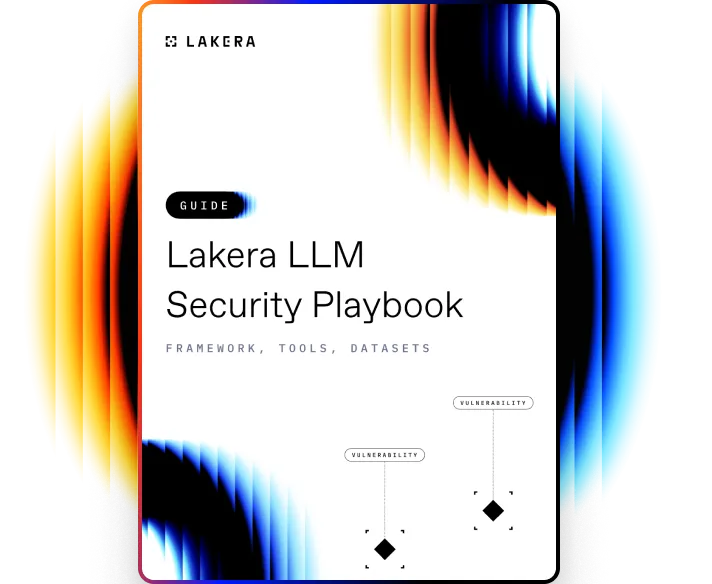
Download this guide to delve into the most common LLM security risks and ways to mitigate them.

untouchable mode.
Lakera Guard protects your LLM applications from cybersecurity risks with a single line of code. Get started in minutes. Become stronger every day.
Several people are typing about AI/ML security. 
Come join us and 1000+ others in a chat that’s thoroughly SFW.