AI Risk Management: Frameworks and Strategies for the Evolving Landscape
Learn the essential AI risk management frameworks for responsible AI development. Understand regulations, mitigate risks, and build trustworthy AI systems.
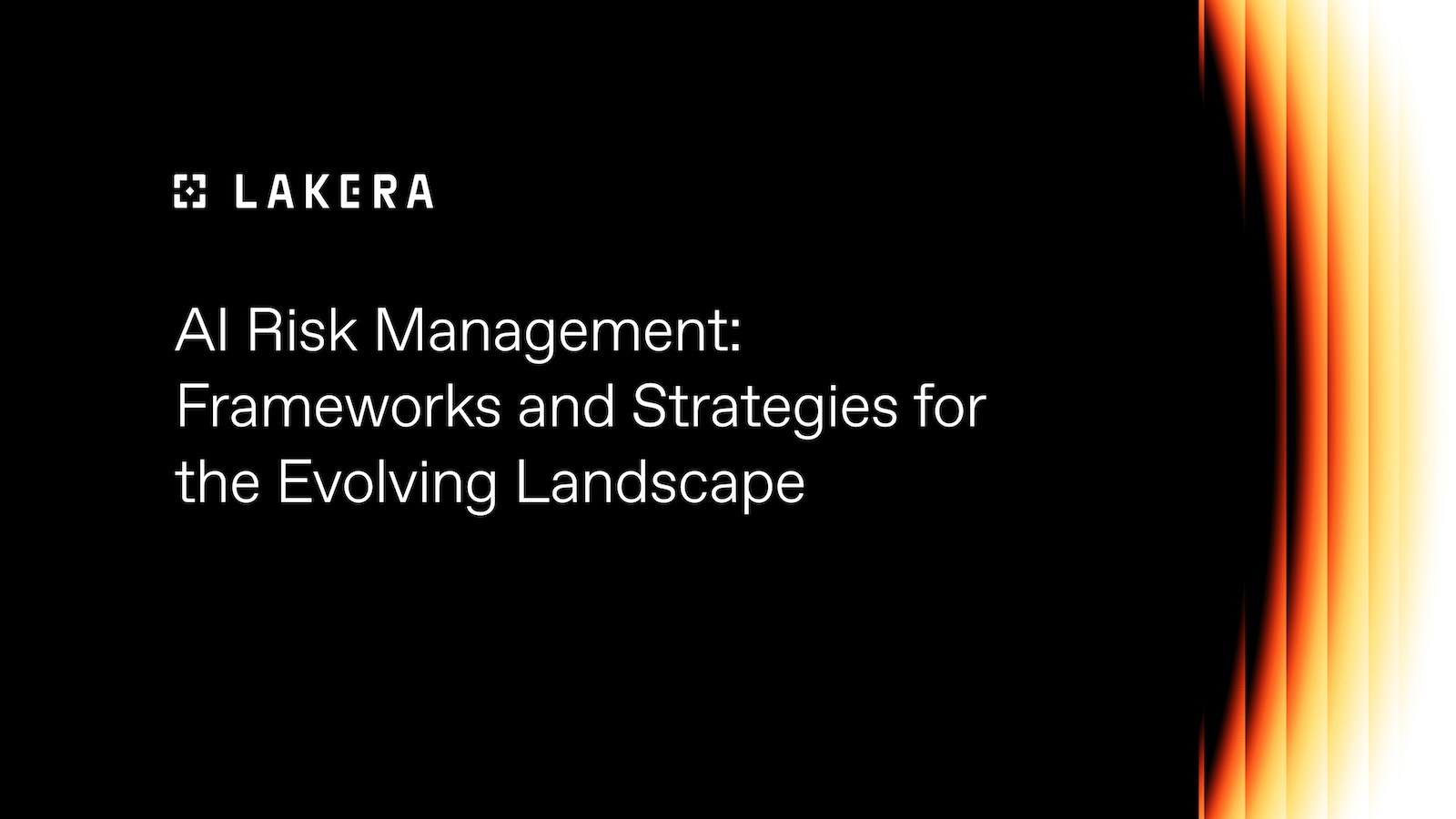
Learn the essential AI risk management frameworks for responsible AI development. Understand regulations, mitigate risks, and build trustworthy AI systems.
Download this guide to delve into the most common LLM security risks and ways to mitigate them.
In-context learning
As users increasingly rely on Large Language Models (LLMs) to accomplish their daily tasks, their concerns about the potential leakage of private data by these models have surged.
[Provide the input text here]
[Provide the input text here]
Lorem ipsum dolor sit amet, consectetur adipiscing elit. Suspendisse varius enim in eros elementum tristique. Duis cursus, mi quis viverra ornare, eros dolor interdum nulla, ut commodo diam libero vitae erat. Aenean faucibus nibh et justo cursus id rutrum lorem imperdiet. Nunc ut sem vitae risus tristique posuere.
Lorem ipsum dolor sit amet, Q: I had 10 cookies. I ate 2 of them, and then I gave 5 of them to my friend. My grandma gave me another 2boxes of cookies, with 2 cookies inside each box. How many cookies do I have now?
Title italic
A: At the beginning there was 10 cookies, then 2 of them were eaten, so 8 cookies were left. Then 5 cookieswere given toa friend, so 3 cookies were left. 3 cookies + 2 boxes of 2 cookies (4 cookies) = 7 cookies. Youhave 7 cookies.
English to French Translation:
Q: A bartender had 20 pints. One customer has broken one pint, another has broken 5 pints. A bartender boughtthree boxes, 4 pints in each. How many pints does bartender have now?
Lorem ipsum dolor sit amet, line first
line second
line third
Lorem ipsum dolor sit amet, Q: I had 10 cookies. I ate 2 of them, and then I gave 5 of them to my friend. My grandma gave me another 2boxes of cookies, with 2 cookies inside each box. How many cookies do I have now?
Title italic Title italicTitle italicTitle italicTitle italicTitle italicTitle italic
A: At the beginning there was 10 cookies, then 2 of them were eaten, so 8 cookies were left. Then 5 cookieswere given toa friend, so 3 cookies were left. 3 cookies + 2 boxes of 2 cookies (4 cookies) = 7 cookies. Youhave 7 cookies.
English to French Translation:
Q: A bartender had 20 pints. One customer has broken one pint, another has broken 5 pints. A bartender boughtthree boxes, 4 pints in each. How many pints does bartender have now?
Artificial intelligence (AI) has transformative potential, but as its capabilities grow, so does the need for effective AI risk management. This field focuses on identifying and mitigating the unique risks associated with the development and use of AI systems. It's distinct from AI for risk management, which involves using AI tools to enhance risk assessment in various sectors like finance or healthcare.
Understanding AI risk management frameworks is crucial for businesses and policymakers. These frameworks provide guidance on addressing the technical, ethical, and societal challenges posed by AI, ensuring responsible and beneficial innovation. Key players like NIST, ISO/IEC, and the European Union have created comprehensive frameworks to manage these risks.
The rapid evolution of AI technologies makes AI risk management an ongoing process. New vulnerabilities and ethical concerns emerge alongside new capabilities, requiring continuous adaptation and vigilance.
In this article, we'll delve into these essential AI risk management frameworks:
{{Advert}}
What are AI Risk Management Frameworks?
AI risk management frameworks provide structured guidelines for identifying, assessing, and mitigating the diverse risks associated with AI systems. They help organizations take a systematic approach to addressing these challenges, rather than relying on ad-hoc or reactive measures.
The best AI risk management frameworks recognize that there's no one-size-fits-all solution. Different industries and applications face unique sets of risks and require tailored approaches. A framework that works well for a self-driving car company might be less applicable for a healthcare organization using AI for diagnostics.
Several key frameworks offer guidance for responsible AI development and deployment:
In the following sections, we'll delve deeper into each of these frameworks, exploring their core principles and practical applications.
The field of AI risk management offers a diverse toolkit for organizations seeking to mitigate the potential harms associated with AI systems.
Frameworks like the NIST AI RMF provide adaptable structures, while global standards like ISO/IEC 23894 promote consistency and transparency. New legal frameworks, such as the EU AI Act, demonstrate the increasing regulatory attention focused on responsible AI use.
Understanding these frameworks is essential for developing and deploying AI in a way that benefits both businesses and society.
The National Institute of Standards and Technology (NIST) AI Risk Management Framework (AI RMF) offers a flexible and comprehensive approach for organizations to address AI-related risks.
Developed through extensive collaboration among industry, academia, and government, the AI RMF emphasizes the importance of building trustworthiness into AI systems throughout their lifecycle.
Alongside the framework itself, NIST provides a companion Playbook. This dynamic resource offers practical guidance for implementing the AI RMF's core functions, allowing for community contributions of best practices and case studies.
The AI RMF revolves around four key functions:
Crucially, the AI RMF is designed to evolve alongside technological advancements and best practices. Voluntary adoption promotes flexibility, allowing organizations to tailor its implementation based on their sector, risk tolerance, and goals.
ISO/IEC 23894:2023 is an international standard specifically designed for AI risk management.
Published in 2023, it provides detailed guidance for organizations across all sectors on identifying, assessing, and mitigating risks associated with the development and use of AI.
The standard offers specific guidance on navigating the complexities of the AI lifecycle.
ISO/IEC 23894 builds upon the established principles of ISO 31000:2018, a broader international risk management standard. This ensures consistency with proven practices while tailoring its approach to uniquely address the challenges posed by AI technologies.
The standard recognizes the specific complexities of managing risks throughout the AI lifecycle.
Annex C of ISO/IEC 23894 provides a valuable tool with its comprehensive mapping of risk management processes across the stages of AI development and deployment. This helps organizations identify where risk mitigation strategies should be applied at each phase.
**It's worth noting that large tech companies like Google play an active role in developing and adapting AI risk management frameworks. Google's Secure AI Framework is a prime example, demonstrating how these principles can be translated into practical, company-specific guidelines for building safe and reliable AI systems.**
ISO/IEC 23894 emphasizes the need for ongoing risk management and continuous adaptation to the evolving landscape of AI technologies.
Its comprehensive approach makes it a valuable resource for organizations seeking to develop and deploy AI responsibly.
The EU AI Act is a landmark piece of legislation designed to regulate artificial intelligence within the European Union. With its focus on promoting safe AI, protecting fundamental rights, and providing legal certainty, the Act has the potential to shape the global AI landscape.
One of the EU AI Act's key objectives is to create harmonized rules across all member states. This aims to establish a single market for trustworthy AI applications, prevent regional differences in regulation, and avoid fragmented standards that could hinder innovation.
The EU AI Act introduces a risk-based categorization system for AI applications, including:
This risk-based approach is crucial for organizations developing or deploying AI systems. It provides a clear framework for aligning their practices with the Act's requirements, ensuring compliance and fostering a responsible approach to AI.
While the EU AI Act directly applies to companies and organizations within the European Union, its influence is likely to extend beyond EU borders.
Multinational companies and others seeking to access the EU market will likely need to align their AI risk management practices with these standards, potentially establishing a global benchmark for responsible AI development.
**💡 Pro Tip: Discover Lakera’s AI governance cheat sheet: EU vs US AI Regulations in a Nutshell**
McKinsey & Company champions a proactive and systematic approach to AI risk identification and mitigation.
Their framework emphasizes integrating legal, risk management, and data science teams from the earliest stages of AI development, fostering an environment where AI models align with both business goals and ethical and regulatory requirements.
McKinsey's approach centers around a structured plan for identifying and prioritizing AI-related risks.
This involves integrating risk management into every stage of the AI lifecycle, ensuring proactive consideration of potential harms and mitigation strategies.
Crucially, McKinsey highlights the importance of collaboration between business, legal, and technical teams. This "tech trust team" approach ensures a comprehensive understanding of potential risks, from legal and ethical implications to technical vulnerabilities.
McKinsey's framework outlines six major categories of AI risk:
Each risk type carries specific considerations. For example, a healthcare AI system handling sensitive patient data will have heightened privacy and security concerns compared to an AI-powered chatbot.
McKinsey guides organizations in creating a detailed catalog of AI risks relevant to their specific applications.
This catalog informs impact assessments, allowing for the prioritization of risks based on their potential for harm. Mitigation strategies can then be tailored to address the most significant issues. Continuous reassessment is essential to account for evolving technologies and new risks that may emerge.
Lakera takes a specialized approach to AI risk management, focusing on the unique security challenges posed by Large Language Model (LLM) based systems. This approach aligns with broader AI security frameworks while offering tailored solutions for a rapidly evolving technological landscape.
We understand the importance of grounding our approach in recognized AI risk categories.
This is why our solutions address key areas outlined in the OWASP Top 10 for LLM Applications, ensuring a comprehensive approach to security threats. Additionally, by proactively targeting risks highlighted by the ATLAS framework, we commit to staying ahead of emerging adversarial AI techniques.
Lakera offers a powerful suite of tools specifically designed to secure AI systems:
Lakera Guard: This specialized tool fortifies AI applications leveraging LLMs. It defends against a wide range of current and future cyber threats, providing organizations with a robust layer of protection.
Lakera Red: This AI red-teaming solution stress-tests LLM-based applications, exposing vulnerabilities before they can be exploited. The adversarial approach proactively identifies potential attack vectors, driving continuous improvement in AI system security.
By focusing on the specific challenges of LLMs, Lakera provides a level of specialized expertise that complements broader AI risk management frameworks.
Our commitment to both defense (Lakera Guard) and proactive testing (Lakera Red) is key to building secure and trustworthy AI systems.
Responsible AI development requires addressing technical risks alongside ethical and societal concerns. Frameworks like the NIST AI RMF, ISO/IEC 23894:2023, the EU AI Act, McKinsey, and Lakera's approach all address this complexity in different but complementary ways.
Framework Highlights:
AI risk management is an ongoing process. Frameworks must evolve as technologies advance, and organizations must constantly adapt their practices.
Key challenges ahead include addressing increasingly complex AI systems, managing algorithmic biases, and ensuring the security of AI applications across industries. Proactive risk management, collaboration, and a commitment to ethical AI development will be essential to unlocking the full potential of AI while mitigating its risks.
Download this guide to delve into the most common LLM security risks and ways to mitigate them.
Get the first-of-its-kind report on how organizations are preparing for GenAI-specific threats.
Compare the EU AI Act and the White House’s AI Bill of Rights.
Get Lakera's AI Security Guide for an overview of threats and protection strategies.
Explore real-world LLM exploits, case studies, and mitigation strategies with Lakera.
Use our checklist to evaluate and select the best LLM security tools for your enterprise.
Discover risks and solutions with the Lakera LLM Security Playbook.
Discover risks and solutions with the Lakera LLM Security Playbook.
Subscribe to our newsletter to get the recent updates on Lakera product and other news in the AI LLM world. Be sure you’re on track!
Lakera Guard protects your LLM applications from cybersecurity risks with a single line of code. Get started in minutes. Become stronger every day.
Several people are typing about AI/ML security. Come join us and 1000+ others in a chat that’s thoroughly SFW.